In an era dominated by the relentless march of technology, the landscape of modern software is evolving at an unprecedented pace. From artificial intelligence and machine learning to blockchain and quantum computing, the digital realm is bursting with innovation. In this article, we embark on a journey through the byte-sized brilliance that shapes the cutting edge of technology and software, exploring the trends, challenges, and promises that define our digital future.
- The Rise of Artificial Intelligence (AI):
Artificial Intelligence has transcended its sci-fi origins to become an integral part of our daily lives. From virtual assistants and recommendation algorithms to autonomous vehicles, AI is reshaping industries and revolutionizing how we interact with technology. The ability of machines to learn, adapt, and make decisions is propelling us into an era where intelligence extends beyond human capabilities.
- Machine Learning and Data-driven Insights:
At the heart of AI lies machine learning, a field that empowers systems to learn from data. As the volume of data continues to explode, the importance of deriving meaningful insights from this information becomes paramount. Machine learning algorithms analyze vast datasets, uncovering patterns and trends that drive innovation in fields as diverse as healthcare, finance, and entertainment.
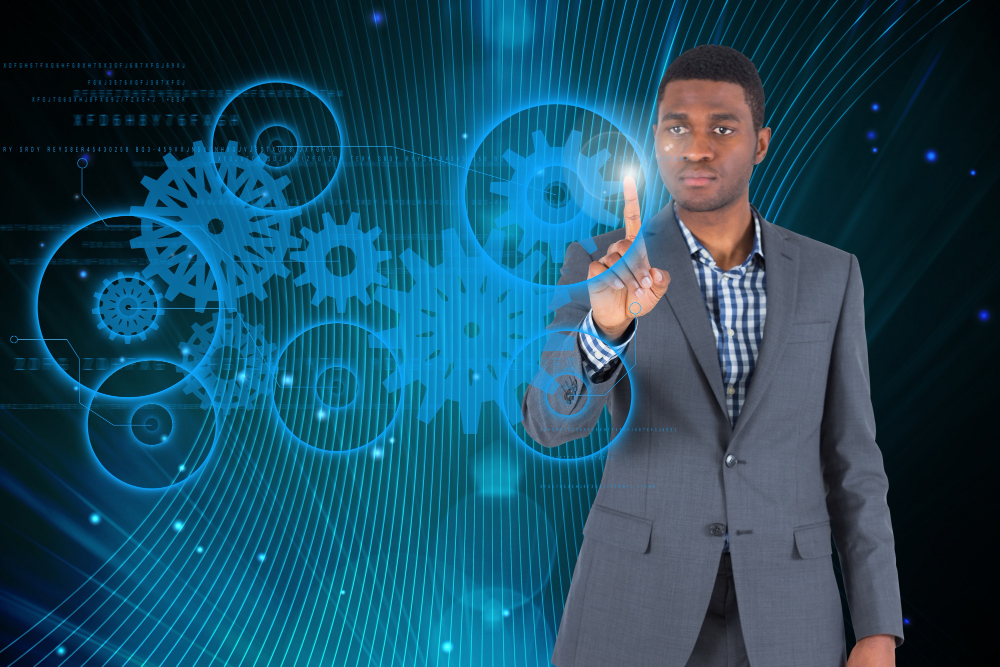
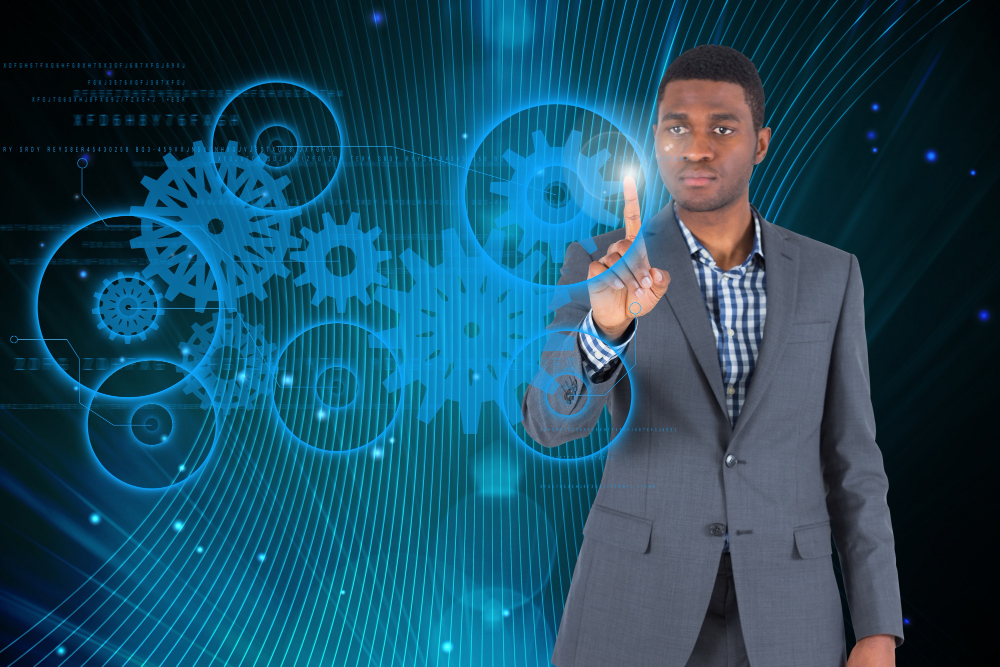
Here are key aspects of Machine Learning and Data-driven Insights:
- Definition:
- Machine Learning (ML): ML is a subset of artificial intelligence that involves the development of algorithms and statistical models enabling computer systems to perform a task without explicit programming. ML systems learn from data, improving their performance over time.
- Data-driven Insights: This refers to the process of deriving meaningful information and knowledge from large sets of data. Insights are generated by analyzing patterns, trends, and correlations within the data.
- Data Collection and Preprocessing:
- The success of ML models depends on the quality and quantity of data. Data collection involves gathering relevant information, while preprocessing involves cleaning, transforming, and organizing the data for analysis.
- Types of Machine Learning:
- Supervised Learning: Involves training a model on a labeled dataset, where the algorithm learns to map input data to a target output.
- Unsupervised Learning: Involves training a model on an unlabeled dataset, allowing the algorithm to discover patterns and relationships within the data.
- Reinforcement Learning: Involves training a model to make sequences of decisions by providing feedback in the form of rewards or penalties.
- Applications:
- Predictive Analytics: ML models are used to predict future outcomes based on historical data, such as sales forecasts, customer behavior, and market trends.
- Image and Speech Recognition: ML algorithms can recognize patterns in images and speech, enabling applications like facial recognition and voice assistants.
- Natural Language Processing (NLP): ML is used to understand and generate human language, facilitating applications like chatbots, sentiment analysis, and language translation.
- Data-driven Decision Making:
- Organizations leverage data-driven insights to make informed decisions. ML models can analyze vast amounts of data quickly, providing valuable information for strategic planning and operational improvements.
- Challenges:
- Data Quality: Poor data quality can lead to inaccurate predictions and insights.
- Interpretability: Understanding how ML models arrive at specific conclusions can be challenging, particularly in complex models like neural networks.
- Ethical Considerations: ML systems may inadvertently perpetuate biases present in the training data, raising ethical concerns.
- Future Trends:
- Explainable AI (XAI): Efforts to make ML models more transparent and understandable.
- Edge Computing: ML models deployed on edge devices for real-time processing.
- Automated Machine Learning (AutoML): Streamlining the ML model development process for non-experts.
Machine Learning and data-driven insights are transforming industries by enabling automation, improving decision-making processes, and uncovering valuable patterns within vast datasets. Continued advancements in these fields are expected to shape the future of technology and business.
- Blockchain: A Decentralized Revolution:
Blockchain technology, synonymous with cryptocurrencies like Bitcoin, is now stepping into new realms. Beyond digital currencies, blockchain promises transparency, security, and decentralization. From supply chain management to voting systems, the distributed ledger technology is challenging traditional norms and redefining trust in the digital age.
- Quantum Computing: Unleashing Unprecedented Power:
Quantum computing, with its mind-bending principles of superposition and entanglement, is on the brink of revolutionizing computation. While still in its infancy, quantum computers have the potential to solve complex problems at speeds unimaginable with classical computers. The race to harness this quantum power is underway, promising breakthroughs in cryptography, optimization, and scientific simulations.
- The Cloud and Edge Computing:
The cloud has become an omnipresent force, enabling businesses and individuals to access computing resources remotely. As demands for low-latency and real-time processing grow, edge computing is emerging to complement the cloud. This hybrid approach ensures that data is processed closer to the source, reducing latency and enhancing the efficiency of applications, particularly in the Internet of Things (IoT) landscape.
- Challenges on the Horizon:
Despite the promises, the landscape of modern technology is not without its challenges. Privacy concerns, ethical considerations in AI, and the environmental impact of data centers are pressing issues that demand attention. Striking a balance between innovation and responsibility is crucial as we navigate the complexities of a digital future.